Cynthia RudinNobel Conference 57
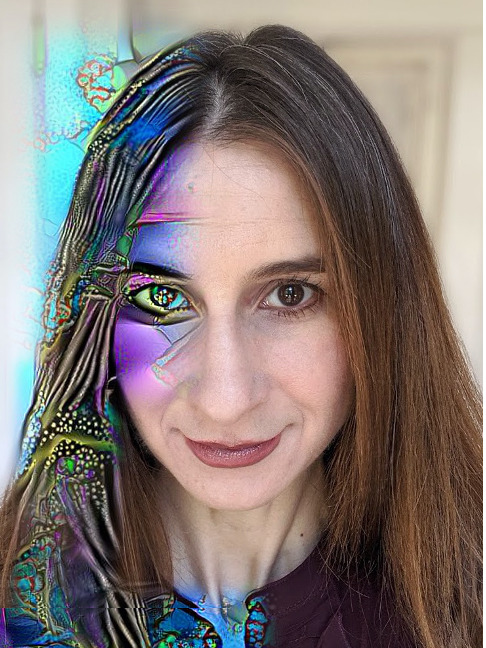
Machine learning, the branch of artificial intelligence focused on learning from data, influences every one of us almost every day. Machine learning models lie behind your internet navigation, predicting what you might want to see or purchase. They underpin loan decisions. They're embedded in speech recognition software. They enable mail sorters and ATM machines to recognize handwritten digits, and they make possible the vision systems of self-driving cars.
While machine learning predictions have largely been used in low-stakes decisions such as advertising or labeling images on social media, they are starting to be used in contexts in which incorrect decisions could be disastrous: health care decisions involving critically ill patients, and criminal justice.
Machine learning models are often "black boxes." That term means either that a model's computations are so complex that humans could not possibly understand them, or that they are proprietary--the exclusive property of a company or other entity, which they keep secret. While black box models may not be particularly problematic when used in low-stakes decisions such as advertising, their use in high-stakes decisions has become dangerous.
Cynthia Rudin studies and advocates instead for the use of interpretable models--machine learning programs that humans can understand, but that can be as efficacious as their black box counterparts. Among the collaborative projects on which she works, she led the first major effort to maintain a power distribution network with machine learning (in New York City), and she developed algorithms for crime series detection, which allow police detectives to find patterns of housebreaks. She also works with neurologists to predict subclinical seizures in patients within the intensive care units of hospitals.
Cynthia Rudin is a professor of computer science, electrical and computer engineering, and statistical science at Duke. She is also the principle investigator of the Prediction Analysis Lab, the focus of which is interpretable machine learning. Rudin is a fellow of both the American Statistical Association and the Institute of Mathematical Statistics. She is a three-time winner of the INFORMS Innovative Applications in Analytics Award, and was named as one of the "Top 40 Under 40" by Poets and Quants in 2015, and was named by Businessinsider.com as one of the 12 most impressive professors at MIT in 2015. She holds a PhD in applied and computational mathematics.